In recent years, renewable energy has emerged as a pivotal force in combating climate change and transitioning towards a sustainable future. Alternative energy sources, such as solar, wind, geothermal or biomass energies, differ from fossil fuels in their diversity, abundance, potential for use anytime and anywhere, and more negligible environmental impact. Solar photovoltaic energy consistently takes the lead among renewable energies: a clean and accessible resource is expected to account for about 60% of the renewable growth over the next five years.
As the world seeks to harness the potential of solar energy, data analytics in optimising its generation and efficiency becomes increasingly vital. By analysing and interpreting vast amounts of data, data analytics in solar energy allows companies to optimise power generation through real-time monitoring of solar components, predictive maintenance, accurate energy forecasting, fault detection, energy consumption analysis and cost reduction.
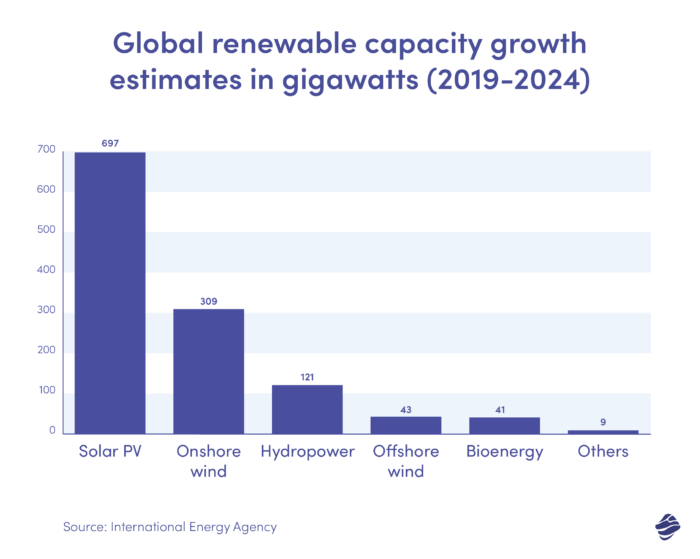
What is solar energy data analysis? Which data analytics applications will reshape the industry in the years to come? How to leverage innovation for business development in the photovoltaic industry? Let’s explore the transformative power of data science consulting in unlocking solar power’s growth potential.
What Is Data Analytics In Solar Energy
Data analytics in solar energy involves data collection, analysis, and interpretation techniques to extract meaningful insights, identify patterns, and make data-driven decisions. Usually, it is applied to various aspects of solar energy, including system performance, energy generation, financial analysis, maintenance optimisation, or grid integration. It involves leveraging data from multiple sources, such as:
- solar panels,
- sensors,
- weather stations,
- smart metres,
- and operational systems.
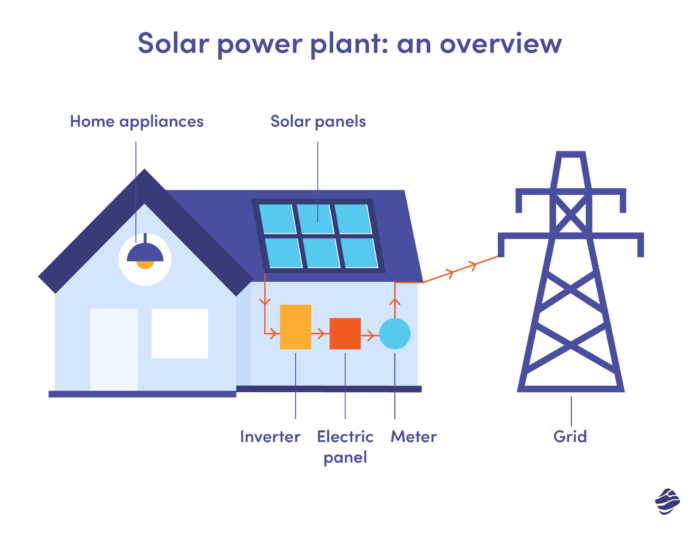
Access to a large amount of data and its proper processing allows solar energy businesses to improve solar power systems’ performance, efficiency, and overall management. But to make it happen, it is crucial first to select appropriate analytical processes and techniques that address the company’s needs.
Solar Energy Data Analysis Techniques
Various analytical techniques are employed in the renewable energy industry, with the primary objective of detecting faulty equipment and irregular energy production, optimising configurations to maximise energy output, and assessing solar energy potential for specific regions. The most popular solar energy data analysis techniques include:
- Machine Learning: Machine learning techniques are used for solar energy generation forecasting, anomaly detection, and predictive maintenance. ML algorithms can analyse historical data on solar irradiance, weather patterns, and system performance to make accurate predictions and optimise operations.
- Time-Series Analysis: Time-series analysis allows solar companies to dive deeply into the historical data collected over time, such as energy generation, weather conditions, and equipment performance. It helps identify patterns, trends, and seasonality, enabling stakeholders to make predictions, detect anomalies, and optimise system performance.
- Statistical Analysis: Statistical techniques such as regression analysis, hypothesis testing, and correlation analysis are applied to understand the relationships between variables, estimate parameters, and assess the significance of factors impacting solar energy generation and system performance.
- Data Visualisation: Visual representations such as charts, graphs, and maps make it easier to display patterns, trends, and performance metrics – helping decision-makers to understand complex data and communicate insights effectively.
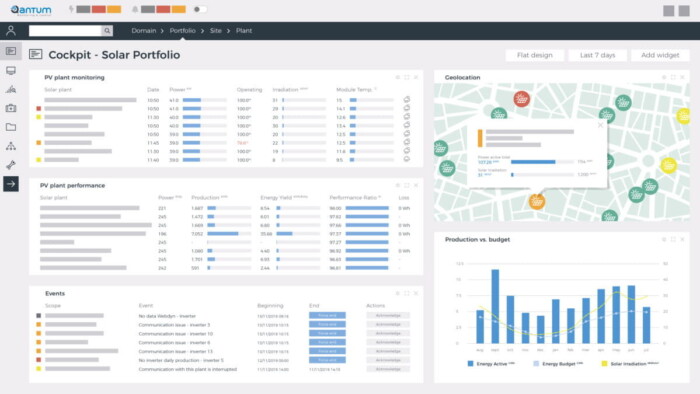
- Predictive Modelling: Predictive modelling employs historical data and statistical techniques to predict future events or outcomes. The solar power industry uses predictive models for energy generation forecasting, system performance optimisation, financial analysis, and risk assessment.
- Big Data Analytics: With the increasing volume of data generated by solar power systems, big data analytics techniques (e.g. distributed computing, parallel processing, and scalable algorithms) allow businesses to handle and analyse large datasets.
- Geographic Information Systems (GIS): GIS techniques combine spatial data with solar energy data to analyse the suitability of locations for solar installations. GIS tools can assess factors such as solar irradiance, shading, land availability, and proximity to infrastructure.
- Pattern Recognition Techniques: Pattern recognition makes it possible to identify meaningful structures. Techniques such as clustering (grouping similar data points), classification (categorising data into predefined classes), and anomaly detection (identifying abnormal patterns) enable stakeholders to optimise system performance, detect irregularities, make accurate predictions, and improve decision-making.
Stages Of Data Analytics In Solar Energy Industry
To optimally use the potential of the collected data to make informed business decisions, the process of data analytics in the solar energy sector should involve the following stages:
- Data Collection: Solar energy systems generate a vast amount of data, including solar irradiance, temperature, voltage, current, energy production, and more. Data analytics involves collecting this data from different sources and ensuring its accuracy, completeness, and reliability.
- Data Integration: Integrating data from multiple sources is crucial for a comprehensive view of solar energy systems. This includes merging data from solar panels, inverters, weather stations, and other relevant sources into a centralised database.
- Data Pre-Processing: Raw data often requires cleaning and transformation before analysis, e.g. removing outliers, handling missing data, data normalisation, and ensuring data consistency to improve the quality and reliability of analyses.
- Performance Monitoring: Data analytics enables real-time monitoring of solar power systems, allowing operators to track energy production, system performance, and efficiency metrics.
- Energy Forecasting: By leveraging historical data, weather patterns, and other relevant factors, data analysts can forecast solar energy production. This helps in grid integration, energy management, and planning activities by predicting solar power generation levels for future timeframes.
- Fault Detection and Diagnostics: Data analytics can identify and diagnose faults, anomalies, or performance issues within solar energy systems. Analysing data from sensors and monitoring systems makes it possible to detect problems like shading, equipment malfunctions, or suboptimal performance, facilitating timely maintenance and troubleshooting.
- Further Optimisation: By analysing performance data, energy consumption patterns, and environmental factors, operators can identify opportunities to improve system efficiency, reduce costs, and enhance overall performance.
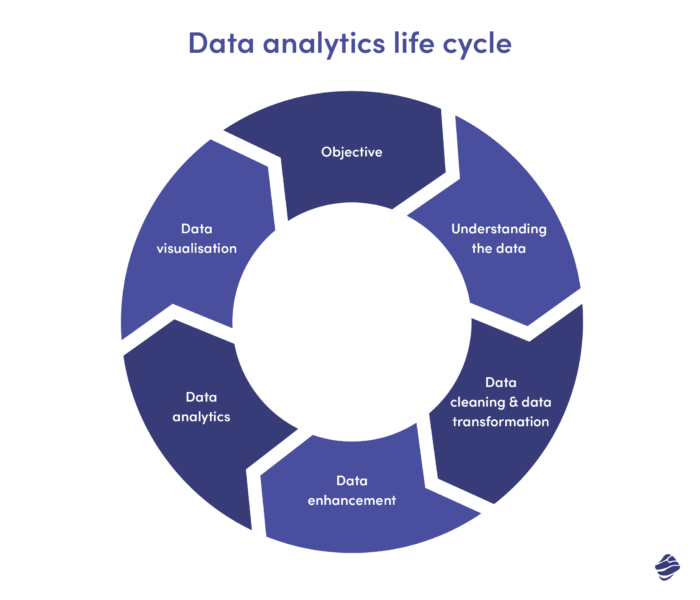
By applying the above data analytics lifecycle, solar power organisations can collect and analyse reliable data, gather meaningful insights, implement data-driven solutions, and continuously monitor and iterate to drive improvement. By paying attention to every solar energy data analysis stage, companies can detect and respond to changes faster than their competition – and maximise data science business benefits.
Applications Of Solar Energy Data Analysis
Data analytics in solar energy poses significant benefits for both utility companies and their customers. By leveraging data analytics software and energy dashboards, energy managers can effectively monitor and minimise grid downtime, accurately predict fluctuations in market demand, optimise asset allocation, and track progress toward environmental goals in real-time. Simultaneously, energy consumers can utilise energy data analytics to pinpoint opportunities for cost savings, align their usage with strategic objectives, and engage with site management to address energy consumption challenges.
Let’s discuss the applications of data analysis in the solar energy industry for companies involved in the construction and distribution of photovoltaic technologies. According to the Solar Energy Technologies Office in the U.S. Department of Energy, data analysis is particularly useful in examining:
- technology costs,
- location-specific competitive advantages,
- policy impacts on system financing,
- detailed levelized cost of energy (LCOE) analyses,
- performance and reliability of solar energy facilities,
- and predicted energy output.
Data Analytics In Solar Energy Enhances Performance Monitoring and Optimisation
Solar energy data analysis allows power operators to monitor the performance of their systems in real time and identify opportunities for optimisation. By analysing data from solar panels and weather conditions, operators can detect faults or underperforming panels and improve overall energy production.
SunPower, one of the leading solar panel manufacturers, implemented a data analytics solution to monitor the performance of their solar installations. The system collects data on individual panels’ performance, identifies issues, and provides real-time alerts to maintenance teams. This resulted in a 10% increase in energy output for their solar installations.
Predictive Maintenance
The solar energy industry often promotes a “install it and forget it” mentality regarding solar panels, which overlooks potential system performance issues. Meanwhile, flaws in design or installation, as well as the accumulation of dirt and debris on panels, can lead to decreased performance. While routine maintenance and monitoring are often neglected, they are crucial for maximising solar system performance and longevity.
Data analytics helps identify maintenance requirements proactively, reducing downtime and increasing the lifespan of solar power systems. By analysing historical performance data and combining it with real-time monitoring, predictive maintenance algorithms can predict when components might fail, enabling operators to schedule maintenance activities efficiently.
According to PV System Predictive Maintenance: Challenges, Current Approaches, and Opportunities research, addressing potential degradation issues in PV systems can be achieved in four main ways: through manual diagnostics, failure modes and effects analysis (FMEA), machine learning & forecasting or by using real-time sensors.
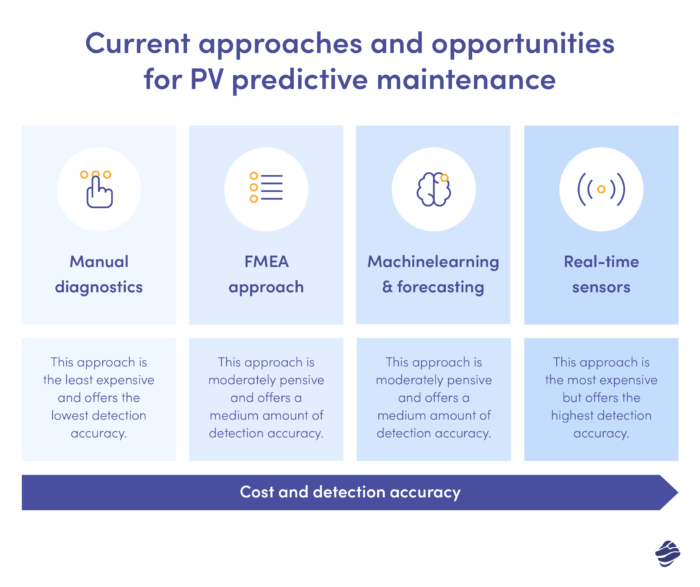
Energy Demand Forecasting
Data analytics in solar energy enables accurate forecasting of energy demand, allowing power operators to adjust their energy generation and distribution accordingly. By analysing historical energy consumption patterns, weather data, and other relevant factors, solar power companies can optimise their energy production and avoid under or overproduction.
The SolarAnywhere suite of solar data services utilises data analytics to provide highly accurate solar irradiance forecasts, helping utilities and solar power operators optimise their energy generation and grid integration.
To avoid energy imbalances and decrease operational risk, independent power producers (IPPs), fleet operators and other solar stakeholders need to be able to predict solar output. Without reliable and accurate solar power forecasting, solar stakeholders may need to make up for unpredicted imbalance with shorter-term sources of power. These short-term power sources tend to be costlier on a per unit basis, lead to financial losses and erode project profitability.
SolarAnywhere SolarAnywhere Forecast
Cost Reduction and Financial Analysis
One of the essential applications of data analytics in solar energy is assisting in financial analysis and cost reduction by optimising system design, improving efficiency, and identifying cost-saving opportunities. Solar power companies can optimise their investments, reduce operational costs, and improve return on investment (ROI) by analysing data on equipment performance, energy production, and financial metrics.
Typical solar technology cost analysis examines the technology costs and supply chain issues for solar photovoltaic (PV) technologies – therefore, it consists of stages such as:
- Manufacturing Cost Analysis,
- Installed System Cost Analysis,
- Levelized Cost of Energy Analysis,
- Supply Chain & Industry Analysis,
- System Operations & Maintenance Analysis.
Each stage leverages data science-based methods, such as analysing performance data on large numbers of PV systems, historical and future cost modelling, and utilising machine learning to analyse data.
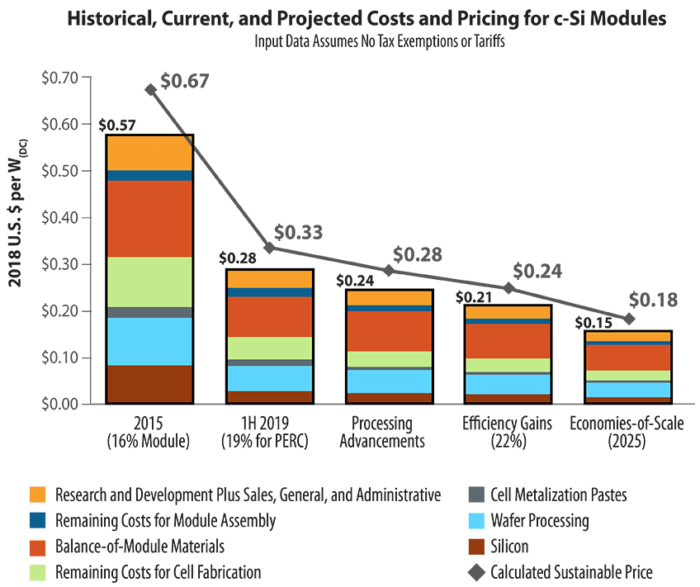
Grid Integration and Stability
Data analytics is crucial in integrating solar power into the electrical grid, ensuring stability and reliability. By analysing data on energy generation, demand, and grid conditions, operators can optimise solar power integration, manage supply and demand fluctuations, and enhance grid stability.
The New York Independent System Operator (NYISO) implemented a data analytics platform to manage integrating renewable energy sources, including solar power. By analysing real-time data on energy generation, weather conditions, and grid load, NYISO optimises the integration of solar power into the grid, ensuring stability and reducing the need for fossil fuel-based backup generation.
The Future Of Data Analytics In Solar Energy Industry
Data analytics has emerged as a game-changer in the solar power industry, revolutionising how companies generate and utilise renewable energy. Solar energy data analysis empowers businesses to anticipate potential issues or failures in solar power systems. It helps to ensure uninterrupted energy production, optimise the allocation of resources such as solar panels, batteries, and inverters, or dynamically adjust energy generation and storage based on demand patterns.
What does the future hold for the renewable energy industry? Firstly, integrating AI and ML algorithms will enhance data analytics capabilities in the solar power sector through even more detailed forecasts and predictions. Both AI and ML are instrumental in addressing the unpredictability of renewable energy by providing accurate weather forecasting, detecting anomalies, and observing patterns nearly in real time.
In the context of the future of data analytics in the solar energy industry, it is also worth remembering the growing importance of the Internet of Things. Constantly improved IoT devices, such as smart metres, sensors, and monitoring systems, can provide real-time data on energy production, consumption, and system performance. This influx of data will fuel advanced analytics algorithms, enabling real-time monitoring, anomaly detection, and predictive maintenance of solar power systems.
Leveraging data analytics in the solar power industry enables companies to unlock the full potential of renewable energy sources by optimising solar power systems’ performance, efficiency, and reliability. It empowers companies to make data-driven decisions, predict energy generation and demand, optimise resource allocation, and enable proactive maintenance. In the context of the solar power industry, leveraging data analytics always involves the intersection of business intelligence vs data science, where actionable insights are derived from data to optimise solar energy systems and drive business success. Ultimately, data analytics drives the growth and scalability of the solar power industry, fostering a more sustainable and resilient energy ecosystem crucial for mitigating climate change and achieving a greener future.