The insurance industry—true to its risk-averse nature—approaches AI adoption with measured caution. Much like crafting a sound life insurance policy, introducing innovation requires careful planning to avoid unexpected consequences and costly surprises.
But even cautious insurers are beginning to see the writing on the wall: automation is no longer a question of if, but when. In fact, nearly 65% of insurance companies plan to invest over $10 million in AI and automation technologies within the next three years.
The boldest players are already reaping the benefits. As the use cases we’ve gathered demonstrate, calculated risks can yield high returns—even in a sector built on security and predictability. From fraud detection to claim triage, insurers integrating AI across various stages of claims processing are unlocking measurable value in record time.
Challenges in traditional claims processing
Not long ago, the typical claims journey looked like this: a claimant would report an incident—say, a car accident or property damage—by filling out detailed forms and attaching supporting documents, usually in paper format. These were then submitted to the insurance company, reviewed by adjusters, and followed by manual assessments and back-and-forth communication. The entire process could stretch out for weeks or even months, with every missing detail or processing error adding further delays.
That’s not just inefficient—it’s costly. According to Accenture’s 2022 report, poor claims experiences could jeopardize up to $170 billion in insurance premiums over the next five years, as dissatisfied customers look elsewhere.
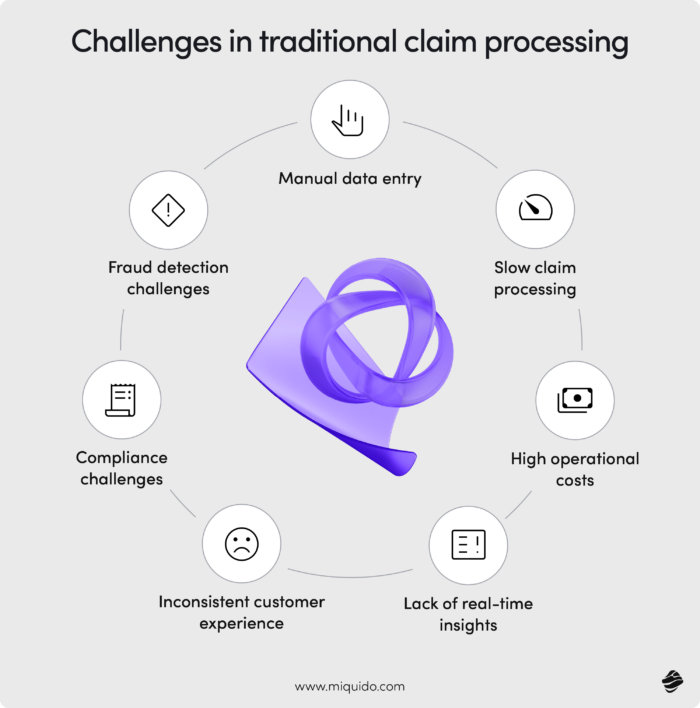
Customer expectations are evolving fast. In the same study, 31% of policyholders reported dissatisfaction with their home and auto insurance claims processes, mainly due to slow settlements and cumbersome closing procedures. Accustomed to AI-powered, seamless digital experiences in banking or entertainment, today’s customers demand the same efficiency and personalization from their insurance providers.
Insurance may not be the first sector that comes to mind when thinking of innovation. But for companies willing to go beyond basic automation, AI in claims processing presents a chance to stand out as industry pioneers. Explore a wide range of use cases and real-world examples of successful implementations to discover how AI can become your competitive advantage.
Claims intake with AI
A mid-sized insurer operating in a European market handles approximately 1,500 claims per day. Each of these cases includes a multitude of data points—each critical for proper resolution. The sheer volume increases the risk of human error in manual processing, leading to delays, complaints, and significant resource strain.
AI streamlines the intake process using natural language processing to extract, classify, and prioritize data with impressive speed. By instantly tagging claims with relevant categories, assigning urgency levels, and routing them to the appropriate departments, AI removes friction from the very first step of the claims journey.
Imagine this: a customer on vacation experiences a minor car accident. In the traditional scenario, they would spend hours filling out forms, calling customer service, uploading photos manually, and waiting days for confirmation. With AI in place, the same customer uses a mobile app to describe the incident verbally, uploads a few photos, and receives automated feedback and next steps within minutes. No long calls, no paperwork, just seamless resolution.
Automating First Notice of Loss (FNOL)
First Notice of Loss (FNOL) is the moment a policyholder reports a loss to their insurer – it initiates the entire claims process. It’s a crucial touchpoint, and the speed and clarity of this first interaction significantly affect customer satisfaction and claim resolution time.
In emergencies, customers don’t want to deal with spreadsheets or fill-in-the-blank forms. They often delay claims simply because the process feels tedious. Instead, AI enables conversational claims filing – customers narrate what happened, and the system transforms this into structured, actionable data.
That’s precisely what Hi Marley achieved with its AI solution. By making FNOL intuitive and human-friendly, the claims handler not only improved user satisfaction but also enhanced operational efficiency on the insurer’s side.
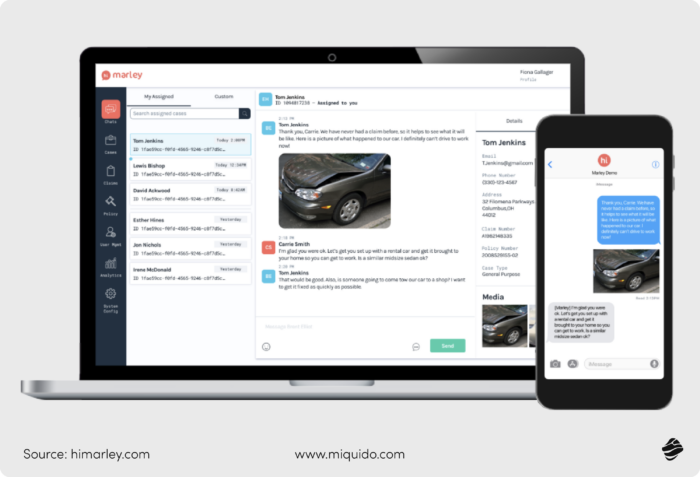
Streamlining data collection and validation
Manually collecting and verifying data from claims eats away at productive hours – hours that underwriters and agents could spend on higher-value tasks. According to Accenture, underwriters lose up to 40% of their time to non-core administrative work, leading to potential efficiency losses ranging from $85 to $160 billion over five years.
AI reduces this burden dramatically. Take Aviva, for example: their sales team needed a mobile-first solution to manage leads, improve customer relationship management, and streamline administrative tasks. A tailored app now supports them in organizing meetings, generating instant reports, and submitting data directly to headquarters – complete with geolocation and real-time notifications. As a result, Aviva not only accelerated internal workflows but also gained better insights into client behavior.
In sectors where insurance structures are particularly intricate, such as marine insurance, AI plays a critical role in simplifying claims. Omni:us’s collaboration with MS Amlin Marine NV illustrates this perfectly. Together, they fully automated the marine claims process and are already planning to expand AI-powered end-to-end automation to high-frequency property and casualty lines.
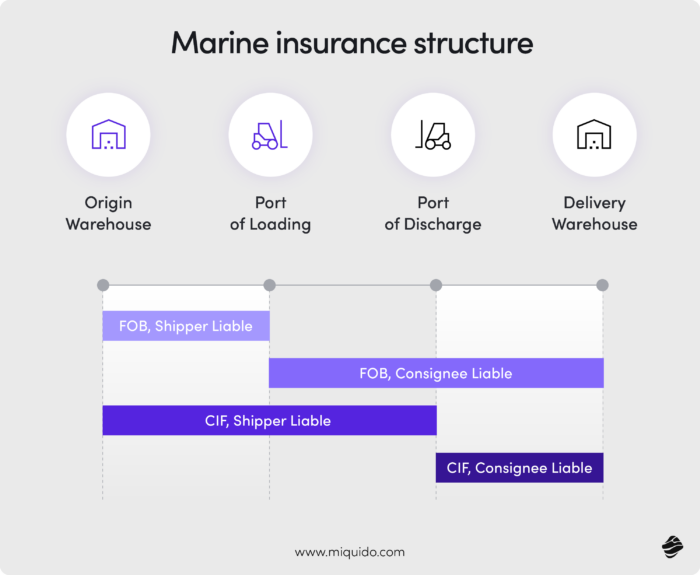
Claims assessment and decision-making
Extracting data is just the beginning – the real challenge lies in what comes next. An insurer needs to assess whether a claim is complete, justified, reliable, and authentic. This evaluation hinges on many interrelated factors, and it’s precisely where traditional manual review starts to show its limits within the broader claims management process.
Take this scenario: a reported repair cost might fall within normal ranges and raise no suspicion on its own. However, when cross-referenced with the type of damage and the vehicle’s age, it suddenly appears excessive, signaling a potential red flag.
AI steps in here as a powerful support system for underwriters. While the final decision still rests with human experts, AI pre-screens claims, organizes relevant and unstructured data, highlights inconsistencies, and presents early-stage insights – allowing underwriters to focus their time and expertise where it matters most. As a result, the claims management process becomes faster, more reliable, and better aligned with client expectations.
Utilizing predictive analytics for faster evaluations
Evaluation time is one of the core KPIs for every insurance provider. The longer it takes to process a claim, the higher the chances a frustrated customer will churn. And while there’s enormous pressure to accelerate claim decisions, doing so manually often compromises quality.
Predictive analytics helps bridge this gap. It enables insurers to evaluate claims faster and more fairly – without cutting corners. Clients benefit from speed and transparency, while insurers streamline their claims operations and boost productivity. In this way, faster claims processing becomes a reality, not just an aspiration, and every underwriter hour saved strengthens the business case for AI in claims management.
Implementing computer vision for damage assessment
Damage assessment based on photo documentation is critical in many claim types—especially in cases of auto accidents, property loss from natural disasters, and water damage in home insurance. Each case comes with dozens of images to review, and when those images begin to look similar, human attention drops. After several hours, accuracy and alertness falter—something the claims management process can no longer afford.
AI doesn’t get tired. Its vision stays sharp whether it’s analyzing the first or the thousandth photo. It can spot anomalies and patterns that a human might miss entirely. Combined with optical character recognition, it can even extract insights from forms, receipts, or license plates embedded in the images—enhancing accuracy at every step.
Imagine this: a claims adjuster manually reviewing bumper damage from an accident. The image looks legitimate—until AI detects that the photo has been reused from a past claim filed under a different license plate. A manual reviewer might overlook that detail, especially under time pressure. AI, on the other hand, flags the image immediately thanks to image-matching algorithms and analysis of unstructured data.
That potential is already being realized by Covea, a leading French auto insurer. In partnership with Tractable, Covea has implemented an AI solution that assesses vehicle damage from photos, drastically reducing claim resolution time. The result? Faster claims processing, improved accuracy, and a smoother experience for the customer—a perfect showcase of AI-driven claims management in action.
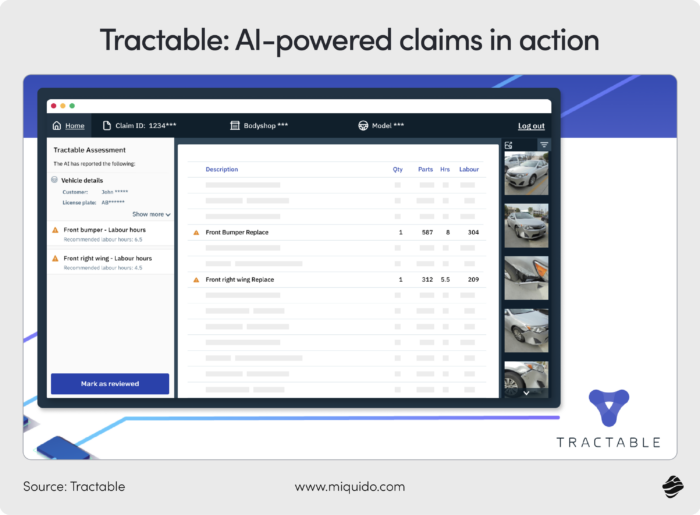
Improving fraud detection and prevention
Insurance fraud is on the rise—and it’s becoming more sophisticated by the year. What’s especially concerning is the growing prevalence of soft fraud. Unlike hard fraud, which involves entirely fabricated events, soft fraud refers to exaggerations or omissions in otherwise legitimate claims—such as overstating the value of stolen items or inflating repair costs. These are particularly difficult to detect, with current detection rates ranging between 20% and 40%, according to a Deloitte report.
While digital tools are critical for attracting and retaining customers, they also open new doors for malicious actors. With the convenience of online submissions and mobile apps, fraudsters might exploit weak verification flows—uploading altered receipts or even using AI-generated deepfakes to fabricate documentation. This forces insurers to rethink how their claims management process handles fraud prevention without compromising on customer experience.
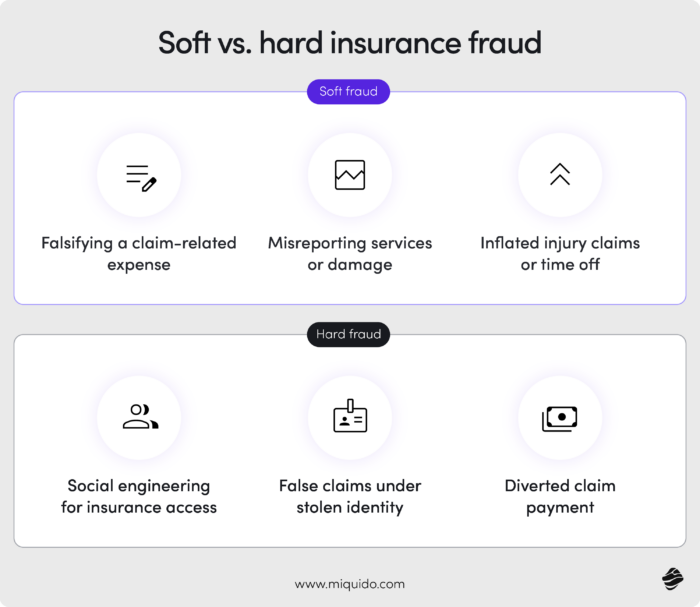
Identifying anomalies with machine learning algorithms
Fraud doesn’t always appear as a blatant lie – it often hides within a web of seemingly unrelated patterns. Imagine a claim filed for fire damage in a vehicle. Alone, the report might not raise alarms. But when combined with inconsistencies in repair history, suspiciously high valuation of the vehicle, and a repeat pattern across related policyholders, the picture changes. Spotting such anomalies is challenging even for seasoned professionals, especially during peak seasons like winter storms or natural disaster events, when claim volumes spike.
Machine learning thrives in this complexity. It can process vast volumes of claims data and learn from historic fraud patterns to detect new threats. Zurich Insurance provides a great example: facing a surge in deepfakes and image tampering, they adopted an AI-driven fraud detection system. The outcome? £78.5 million in fraudulent claims prevented in the UK in 2023. That’s the power of integrating intelligent systems into claims operations.
Enhancing accuracy in fraud detection
When it comes to complex fraud – especially soft fraud – it’s often the nuanced judgment of a human expert that makes the difference. While AI can flag anomalies, it might miss contextual red flags or new tactics not present in its training data. Fraud strategies constantly evolve, and AI systems learn by detecting historical patterns, which leaves gaps when encountering new behaviors.
This is why hybrid models are gaining traction in claims management. Combining the precision and scalability of artificial intelligence with human intuition leads to better results. Think of it as a digital-human partnership: AI handles the heavy lifting- scanning claims, applying optical character recognition, flagging duplicates – while human agents apply contextual reasoning. This synergy boosts accuracy and speeds up evaluations, creating a more resilient claims management system.
Proof? Look at the collaboration between Cognizant and a leading global bank. By deploying an AI-human fraud detection model, they saved $20 million in potential fraud losses.
Optimizing customer communication and experience
The insurance industry operates under unique conditions. Insurance products are usually purchased during specific life events, often as one-off or long-term decisions. Clients aren’t looking for novelty or surprise—they value reliability, trust, and safety. Once a customer places their trust in one provider, they are likely to stay—unless that trust is broken by poor service.
Customer experience is the most critical loyalty factor in insurance. In fact, a 2022 TechSee survey found that 39% of Americans who canceled a contract in the previous two years did so due to poor customer service—and notably, 11% of those cancellations were insurance policies.
Artificial intelligence is helping to rewrite this narrative. With AI-powered solutions, insurers can offer faster claims processing, better communication, and smarter prioritization of urgent cases – all at a significantly lower operational cost. From handling unstructured data to enhancing the entire claims management process, AI optimizes each step in customer interaction.
Deploying AI-powered chatbots for customer support
Few things damage trust like long delays in claims processing. AI chatbots play a key role in accelerating this process. They guide customers through claim submission, extract structured information from narrative descriptions using natural language processing (NLP), and ask clarifying questions to ensure claims reach human agents in a complete and decision-ready form.
A standout example comes from Tryg, one of Scandinavia’s largest non-life insurers. In partnership with Boost.ai, they launched two AI-powered virtual agents – one customer-facing and one for internal use.
Mia successfully automated 80% of over 200,000 customer interactions, providing real-time, accurate support without human intervention. The result? Enhanced customer satisfaction and a drastic reduction in service wait times.
To streamline claims operations and internal support, Tryg also implemented Rosa – an AI assistant for employees. Rosa resolved 97% of internal queries, dramatically reducing calls to back-office teams and boosting operational efficiency across the board.
Personalizing communication through NLP
Today’s customers expect brands to talk to them like individuals, not account numbers. Personalization in insurance isn’t just nice to have – it delivers tangible value. Clients enjoy a smoother journey tailored to their behaviors, while insurers gain new upselling opportunities and stronger relationships.
For global insurers, this means deploying AI that doesn’t just speak the right language—but also understands cultural context and nuance. A perfect example is PT Asuransi Tokio Marine Indonesia (TMI), which partnered with WIZ.AI to launch a conversational voice AI agent—Talkbot.
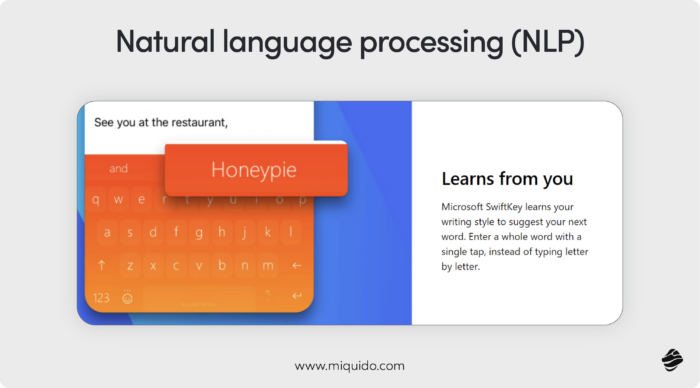
Talkbot feels like a human call center agent—over 95% of callers can’t tell the difference. Using advanced natural language processing and understanding, the bot responds with human-like rhythm, pauses, and tonal variation. It even supports multiple ASEAN languages, making it an invaluable customer communication tool in a region where multilingual precision is essential. In the same way, we optimized the first insurance chatbot on the Polish market, developed in collaboration with PZU.
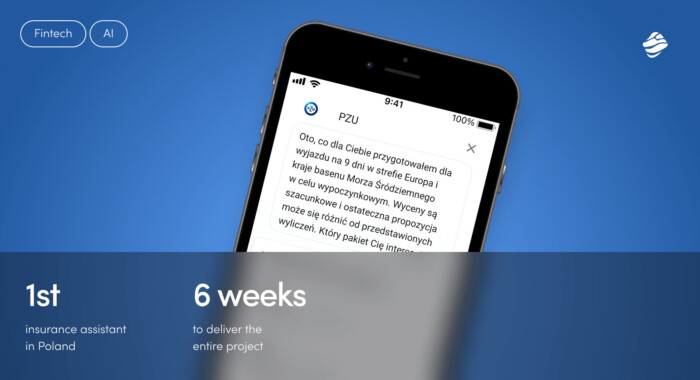
Automating claims settlement and payment
In the race to meet rising customer expectations and improve internal efficiency, insurers are turning to AI not only for claims assessment—but for full-scale automation of the claims settlement and payment process. From first notice of loss to final payout, AI eliminates bottlenecks, reduces manual effort, and ensures a smoother, faster experience for both customers and claims teams.
Reducing settlement times with AI-driven processes
In Austria, where the insurance sector is navigating a wave of digital transformation, traditional insurers are accelerating their AI adoption to keep pace with customer demands for speed and transparency. Allianz has responded to this challenge with a bold move: the deployment of its Insurance Copilot.
Launched to streamline automotive claims workflows, the AI-powered Insurance Copilot handles everything from document processing to decision support. It analyzes damage reports, validates claims against policy data, and flags anomalies—dramatically reducing the need for manual intervention.
The results speak for themselves. Allianz reported significant improvements in both processing speed and claims accuracy, leading to faster settlements and an elevated customer experience. In a competitive market, these enhancements don’t just improve operations—they strengthen customer loyalty.
Ensuring compliance and accuracy in payments
On the other side of the Atlantic, insurers are facing a different but equally pressing challenge: balancing speed with regulatory compliance. In the U.S., accurate and fair claims processing remains a top priority, especially in a landscape where every payout is subject to scrutiny.
Xorbix Technologies tackled this head-on with an AI-driven solution designed specifically to optimize and safeguard the claims management process. By automating claim evaluation, policy matching, and payment validation, Xorbix’s system ensures that every settlement complies with regulatory standards and internal accuracy benchmarks.
Future-proof AI for your claims management
Half of the European insurance companies use AI technology in the non-life business, and within three years, it will be 8 out of 10, according to Eiopa. The unclear regulatory landscape has been holding some of the insurers back, but with the approval of the AI act, they have clear, transparent foundations for their optimizations.
We have already supported insurers like PZU and Aviva – if you are also looking for a tool that will improve your fraud detection capacities, claim processing speed and data integrity, let’s delve into the possibilities.