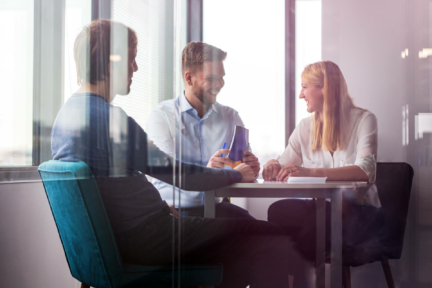
One Shot Learning Definition
-
What Is One-Shot Learning
One-shot learning is advanced machine learning that teaches models to recognise new objects or patterns based on just one or a few examples without needing a large amount of labeled data. One-shot learning is especially useful in real-life situations where getting extensive data can be expensive, time-consuming, or even impossible.
This type of learning is particularly important in fields where obtaining ample labeled data is challenging. It is used in computer vision services, natural language processing, medical imaging, and other domains where learning from very few examples is necessary. However, the success of one-shot learning largely depends on the model architecture, data representation, and task complexity.
Applications Of One-Shot Learning
One-shot learning has numerous applications in various fields. It helps with object and face recognition from minimal examples, handwriting recognition without a large amount of data, and medical diagnostics with limited images. It is also used to identify rare species, detect anomalies in surveillance and finance, and categorise texts in natural language processing. Furthermore, one-shot learning plays a role in art authentication and supports language learning tasks like translation and speech recognition, showing its broad utility in scenarios with scarce data.
See also: AI Agent Definition, AI Hallucination Definition, AI Embeddings Definition,