- Home
- AI Glossary
- Deep Belief Networks Definition
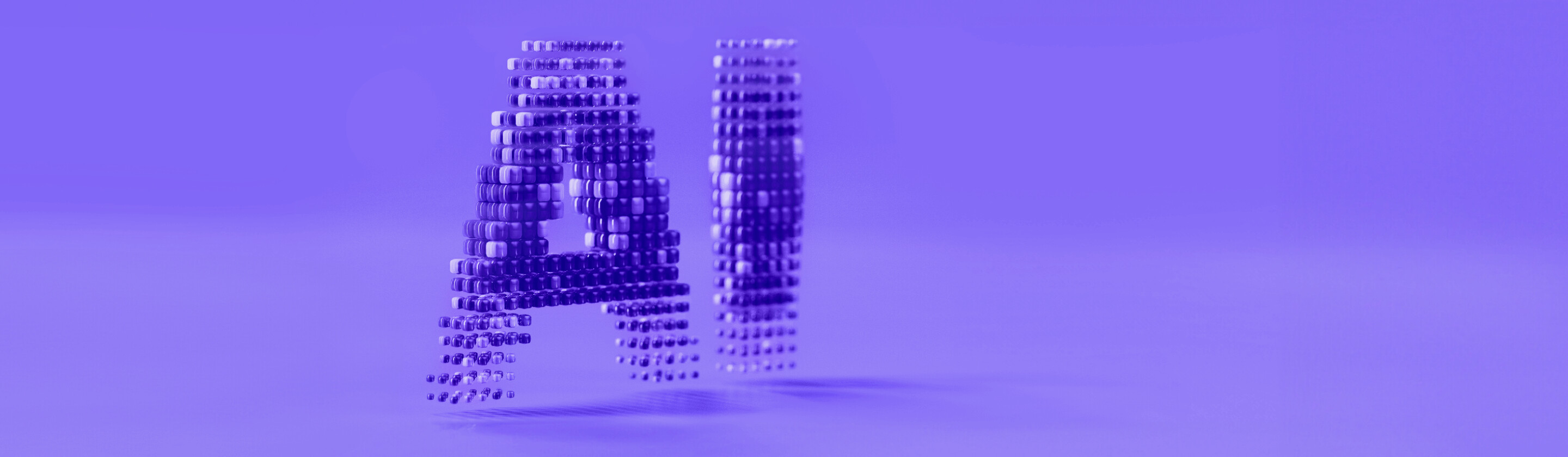
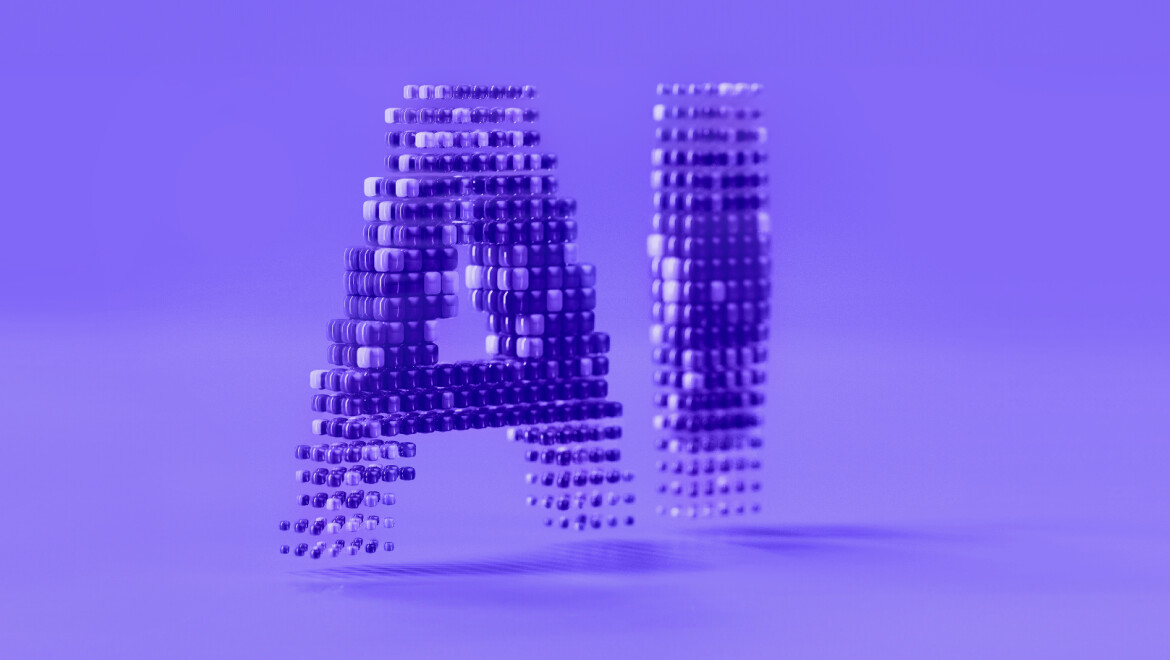
Deep Belief Networks Definition
Deep Belief Networks Definition
What is a Deep Belief Network?
Deep Belief Networks (DBNs) are advanced artificial neural networks that have transformed the field of deep learning. If you’ve encountered this term and are wondering what it means, prepare to embark on a fascinating journey into artificial intelligence development services. A DBN is essentially a stack of unsupervised machine learning models called Restricted Boltzmann Machines (RBMs). These RBMs are linked together to form a deep network that can learn to reconstruct inputs. This makes DBNs powerful tools for feature detection and recognition tasks.
Deep Belief Networks Explained
Recent advancements in deep belief networks (DBNs) demonstrate their versatility in various fields. One notable example is their application in detecting Alzheimer’s disease using multi-omics data, a significant step in medical diagnostics. Additionally, DBNs have been reviewed for their effectiveness in interpreting electroencephalography (EEG) signals, with adaptations tailored to specific medical tasks. This demonstrates the adaptability of DBNs to different types of data and the ongoing efforts to refine their accuracy for practical applications.
Enhancing Deep Belief Networks
Technological improvements have also been made to the training methods of DBNs. A modified approach introduces an adaptive step into the Contrastive Divergence algorithm used during the pre-training phase of DBNs. This innovation aims to overcome challenges in finding the global optimum and enhance the networks’ accuracy and stability. Another significant development is the introduction of a maximum entropy (ME) learning algorithm for DBNs, designed to better handle scenarios with limited training data. This approach contrasts with the traditional maximum likelihood criterion and illustrates the ongoing research to improve DBNs’ learning efficiency and extend their applicability even when data is scarce.
Ready to discover more terms?