- Home
- AI Glossary
- Retrieval Augmented Generation Definition
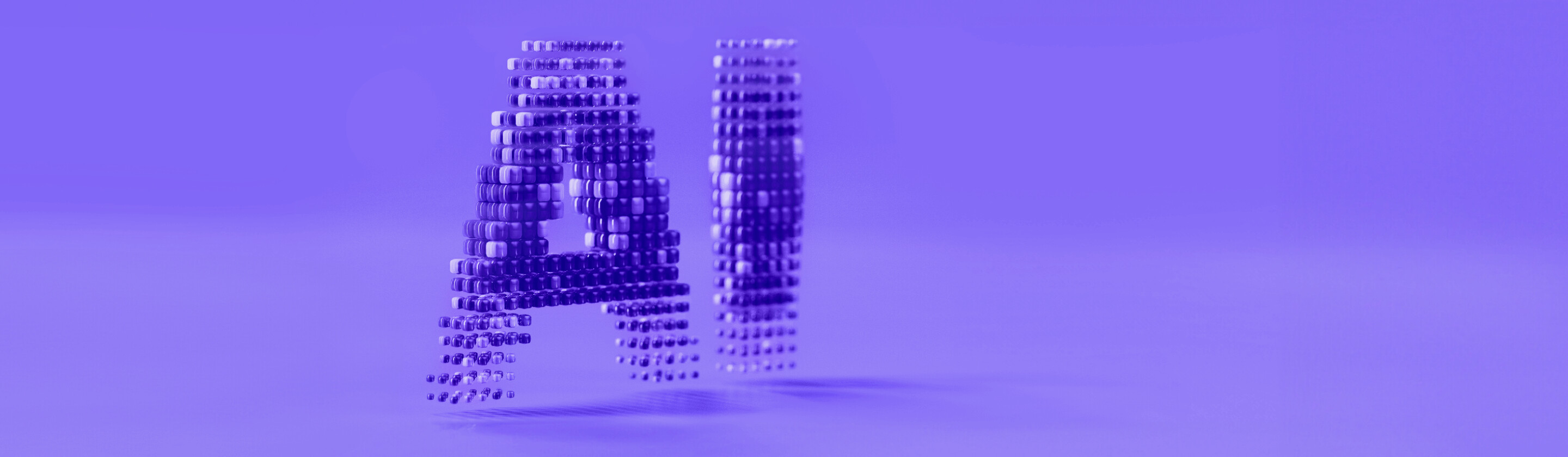
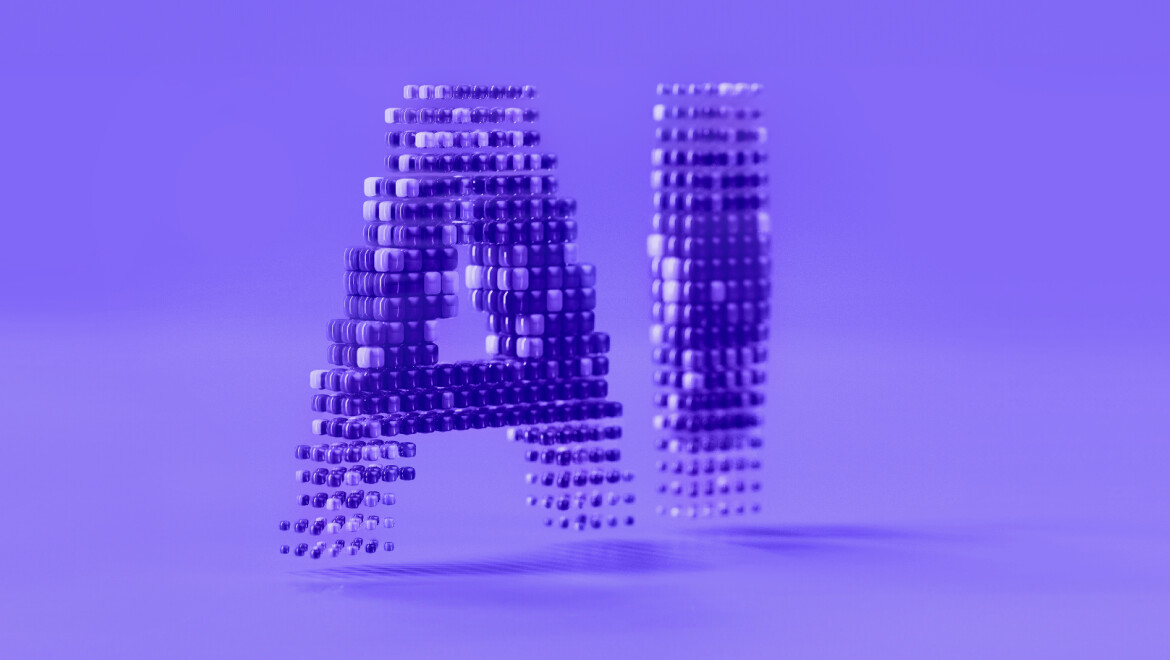
Retrieval Augmented Generation Definition
Retrieval Augmented Generation Definition
Retrieval Augmented Generation (RAG) Explained
Retrieval-Augmented Generation (RAG) is a cutting-edge technique that combines the strengths of information retrieval systems with the generative capabilities of large language models (LLMs) to enhance text generation. This approach allows AI models to pull in external knowledge dynamically during the generation process, enabling more accurate, informative, and contextually rich outputs.
At its core, RAG operates by first querying a large corpus of documents or a database to find relevant information based on the input it receives. This retrieved information is fed into a generative model, such as GPT (Generative Pre-trained Transformer), incorporating this external knowledge into its responses. This two-step process, retrieval followed by generation, allows RAG to produce coherent, contextually relevant outputs deeply enriched by the vast amount of information it can access from external sources.
How does RAG work?
Understanding RAG requires recognizing its role as a bridge between traditional language models, which rely solely on pre-learned information during training, and the dynamic, on-the-fly data retrieval from vast databases or the internet. This method significantly expands the model’s ability to generate responses informed by up-to-date knowledge and facts not contained within its original training data. Therefore, RAG is instrumental in scenarios where the accuracy of the information and the inclusion of the latest data are crucial, such as in answering factual questions, summarizing articles, or even generating content based on recent events.
Where is RAG used?
AI Retrieval-Augmented Generation represents a leap forward in making AI systems more versatile and knowledgeable. By leveraging RAG, developers can create AI applications that are not just parroting pre-learned information but are actively seeking out and incorporating the most relevant and current data. This capability is particularly beneficial in fields like customer support, where providing accurate, up-to-date information is paramount, or in content creation, where referencing the latest trends and data can significantly enhance the quality and relevance of the generated content.
In essence, Retrieval-Augmented Generation marks a significant advancement in the evolution of AI, pushing the boundaries of what machines can understand and produce. By seamlessly integrating external data retrieval into the generation process, RAG-equipped models offer a glimpse into the future of AI: a future where models are not just reactive but proactively informed by a wealth of knowledge, making them more helpful, accurate, and insightful than ever before.
Ready to discover more terms?